The Rise of Artificial Intelligence: The Automation of the Knowledge Worker
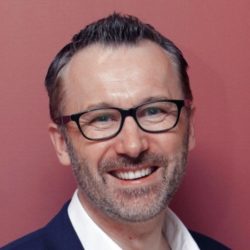
Since Alan Turing posed the question ‘can machines think?‘ in 1950, the concept and perils of artificial intelligence (AI) and intelligent machines has been discussed for decades, mostly in works of science fiction. Yet it’s arrival has been less dramatic and more deceptive, offering recommendations on Amazon, suggesting movies to watch on Netflix, or providing information and answers when we asked for it via voice-activated helpers like Siri, Alexa and Google Assistant.
By Sean Culey
Artificial Intelligence is one of the pivotal technologies of the next wave of technological change. To be deemed as ‘intelligent’, a machine must be able to operate in a multitude of variable conditions, absorb and process a stream of sensory data, find patterns in that data, and be able to determine the appropriate course of action based on the conditions, data and patterns presented. It must be able to learn over time and handle a vast multitude of different variables. Computers are increasingly getting better at handling unexpected situations, and doing so with more and more accuracy. Variations of self-learning algorithms are now routinely embedded in mobile and online services to recommend products and adapt the experience based on our previous choices and preferences. However, we are now starting to see some of the real technologies that will power the AI revolution, especially when it comes to the world of work.
First Movers
AI capabilities such as cognitive computing and machine learning are all advancing behind the scenes. Cognitive computing, the poster child for which is IBM’s cognitive supercomputer named Watson, came to prominence after thrashing the two best competitors ever on the US game show Jeopardy back in 2011. However, whereas Jeopardy was all about providing answers, IBM directed Watson towards the process of discovery, offering Watson’s capabilities to any parties who felt they had a compelling business case for this technology.
The first takers were the healthcare industry, using the cognitive computing power of IBM Watson to analyse large amounts of imaging and text in electronic health records so it could identify traces of malignance. Watson Genomics can create tailored drug recommendations for cancer patients in minutes rather than the weeks it would normally take human doctors. The insurance industry was another place where Watson’s capabilities found a home. In 2017, an insurance firm in Japan replaced thirty-four employees with an IBM Watson based artificial intelligence system, giving the company a return on its investment in under two years.
Watson is not the only AI in town. While AI researchers are busy building smart machines, machine learning experts are striving to make them truly intelligent. Machine learning is the development of an intelligent system that can make its own decisions without being implicitly programmed to do so. Nidhi Chappell, head of machine learning at Intel describes the difference between AI and machine learning as follows; “AI is basically the intelligence – how we make machines intelligent, while machine learning is the implementation of the computing methods that support it. The way I think of it is: AI is the science and machine learning is the algorithms that make the machines smarter. Machine learning is therefore the enabler for AI. If AI is driving the car, machine learning identifies the stop sign and what to do.”
Machine learning is being used to discover patterns and correlations in data, and in doing so powering the development of predictive models and analytics helping companies to provide new offerings in today’s on-demand world. Machine learning powers the new wave ride hailing companies such
as Lyft and Uber, enabling them to determine optimal routes, match rides with drivers, establish ride and wait times and determine prices. In the world of financial services, JP Morgan hit the tech headlines when they announced that they had created a learning machine called COIN (short for Contract Intelligence) that was able to interpret commercial loan agreements in seconds, a task that had previously took their lawyers and loan officers 360,000 hours per year to do. Machine learning is also being used extract and analyse information from unstructured data captured from its customers such as emails, chats, comments, videos and support requests to accurately gauge customer sentiment, such as satisfaction with the service.
Areas which rely heavily on experience and knowledge gained from years of study and in-field practice are suddenly finding themselves being replaced by machines they can process information that it would take a human their entire working life to process in just seconds.
To Whom am I Speaking?
The increasing use of AI and machine learning to power voice recognition systems that understand language and enable conversation via voice rather than text has given rise to the chatbot. The benefits are obvious. Firstly, a chatbot never sleeps, takes a break or is likely to damage your brand by being rude or sarcastic (not yet, anyway). It increases the level of service customers receive by being able to filter out and answer easy questions, while also increasing the response time preventing people from waiting ages listening to looping, soul destroying messages about how important their call is to the company. They can learn by experience, able to answer ever more sophisticated questions relating to the business of the company they support. IBM’s Watson is also being used to power chatbots. Watson Virtual Agent uses its cognitive and deep natural language processing capabilities to provide a conversational self-service experience, providing callers with answers to questions and to appropriately reacting to their requests. Companies like Staples have already utilised this technology to enable people to verbally order goods anytime, anywhere, from any device they prefer. They can also talk to the virtual agent to reorder supplies, track shipments or chat about customer service needs.
A Better Plan. A Better Planner.
The task of planning, especially for consumer facing companies, is becoming untenable. Online shopping continues to explode, and in certain sectors such as apparel, consumer behaviour results in large number of orders being returned because the shopper ordered multiple clothes in multiple sizes, keeping the one outfit they like and returning the rest. These types of demand behavior cause severe headaches for supply chain managers as it has become harder to predict where the product needs to be and when. This creates almost impossible pressure for companies.
Realising the opportunity, supply chain planning companies like ToolsGroup and Quinteq have embedded AI and machine learning into their supply chain optimization software. These solutions create a baseline forecasts by using an algorithm that learns from early levels of consumer demand combined with realistic product output numbers and promotions to accurately forecast upcoming needs and optimize inventory and replenishment plans far better than human planners can. These new forms of data analytics are shaking up multiple industries as machines gain unprecedented capabilities to improve processes that humans have struggled with for decades. Whereas in the past planning was linear, with silo based demand plans, inventory plans, supply plans and even silo exception plans, today they are increasing automatic and event-driven. The ability to plan routes with up-to-date information from smart machines enables more detailed distribution planning, dramatically reducing costs and time to replenishment. An AI planned supply chain could begin to predict how many customers are going to require a product based on when specific sets of criteria are met – for example weather conditions. The ability to make an almost instant prediction based on years of data, to say ‘when a specific set of conditions occurs, x more sales occur’ will prove to be a very powerful differentiator for those companies who can get take advantage of this technology.
Digital Dilbert?
The market for building greater levels of machine intelligence is huge, as is the wealth that will be created because of it. Silicon based AI systems will be able to process and make sense of far more information than our carbon-based brains could ever hope to achieve. What companies are rapidly finding is that AI is cheaper, more efficient, less error-prone and potentially more impartial in its actions than human beings. Many knowledge workers are already doing standard, repetitive work such as contracts reviews, invoice and order processing, mortgage servicing operations and dealing with compliance issues. These can easily be, and are already being, automated.
There is a new sense of urgency to utilise this technology as businesses realise that data has a shelf life and its value diminishes rapidly over time. The more data that is collected, analysed and used, then the greater the economic value it produces. In addition, the economic value of data multiplies when combined with context and right time delivery. Everyone is now in the data business.
Prepare for an AI Future
We have only just stated the AI journey. The technology is in its infancy, yet it is already changing the world around us in profound ways. We haven’t seen anything yet. So how will an increased use of AI affect the way companies liaise with customers and support this relationship with enhanced supply chain functionality?
- When shopping in brick-and-mortar stores, advanced analytics, GPS, computer vision and machine learning algorithms will identify when you are near a store and offer you personalised vouchers, offers and discounts. When inside the store, your purchasing history will be accessed and items suggested and matched to accompany previously bought goods.
- Increased use of store robots who will offer personalized product recommendations made based on data about each customer’s unique interests and buying propensity. Over time, the Amazon Go style experience will expand to other retail shops as well, eliminating checkouts and automatically charging accounts, enhancing the shopping experience.
- At home, demand signals will be immediately received via intelligent machines, prescriptive ordering AI systems, connected smart home devices or by you just placing an order the moment you notice the need. This demand will increasingly include information on the customer’s preferences, location, age and background, and the machine’s current stock levels and consumption data.
- When shopping online, chat bots will increasingly be used to drive intelligent and meaningful engagement with customers, provide customer service and ongoing support to queries to an increasingly level of complexity
- The detailed build-up of data from your viewing and shopping history will enable websites to present an increasingly more tailored list of recommended products, and algorithmic pricing engines will also make personalised, situational pricing offers to each customer.
- These demand signals will be combined and processed by AI and machine learning powered data analytical tools, who constantly adjust replenishment schedules and plans real-time based on the latest consumer data.
- Machine learning based AI systems will be used to provide accurate replenishment forecasts, production and distribution plans, helping to continually establish the optimal cost-to-serve models, stocking profiles and manufacturing schedules. Companies will use AI and machine learning to sharpen analytic algorithms, detect more early warning signals, anticipate trends and have accurate answers before competitors do.
AI tools such as machine learning, chatbots, deep learning and cognitive computing therefore all play a critical part in the next wave of technological change, powering the Personal, Automated and Local supply chain.